As scientists and engineers work to decarbonize our planet, one energy carrier has emerged as a possible substitute for fossil fuels: hydrogen. When used as a fuel, hydrogen does not directly produce any greenhouse gases—it only produces water. Hydrogen can also be used to store and deliver energy produced from other sources.
However, scientists must use electrocatalysts to promote the reactions needed to make hydrogen a viable fuel source. Currently, these catalysts are made from expensive materials like platinum and palladium.
Engineers have tried for decades to find new materials that can provide the same reactions needed for a much lower cost, but such experiments involve a lengthy trial-and-error process in the lab.
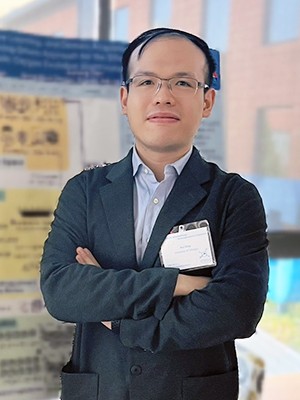
The answer could be found using machine learning, says Rui Ding, an Eric and Wendy Schmidt AI in Science Postdoctoral Fellow co-advised by Prof. Junhong Chen, a leading expert in nanomaterials and water technology, and Prof. Yuxin Chen, who specializes in AI. He should know: he spent five years conducting these trial-and-error experiments for his PhD. But during the COVID-19 pandemic, when he was locked down in his apartment in Wuhan, China, he was unable to return to the lab. So, he switched his focus to AI and machine learning, applying these techniques to materials science research.
Now at the University of Chicago Pritzker School of Molecular Engineering (PME), Ding found that there was a need for a comprehensive review of machine learning techniques that could aid scientists in discovering new electrocatalyst materials. He, Chen, and a group of collaborators reviewed more than 150 papers in this area, evaluating various reactions, potential materials, and applicable machine-learning methods.
The result, published in Chemical Society Reviews, offers a meta-level analysis of the current research landscape, serving as a valuable guide for scientists and industry professionals aiming to advance this area of study.
“If you want to find new electrocatalysts, this paper gives you everything you need to start,” Ding said.
Analysis of each electrocatalyst reaction
The paper offers overviews for each reaction needed to use hydrogen as an energy source. The first two, hydrogen evolution reaction (HER) and oxygen evolution reaction (OER), split a water molecule into hydrogen and oxygen. Electricity is then stored in hydrogen molecules.
The second two, hydrogen oxidation reaction (HOR) and oxygen reduction reaction (ORR), convert hydrogen and oxygen into water. These reactions are necessary for energy release.
For each of these reactions, Ding and his co-authors offer an overview of potential material categories that could be used in electrocatalysts, and offer suggested machine-learning techniques that might help identify the best materials.